Some Observations on the Measurement of Forecast Error and Accuracy
Some obServationS on the meaSurement of forecaSt error and accuracy by ranga Katti In computing percentage error, it is better to use actual as a divisor because we want to know how the forecast deviates from the actual, not how the actual deviates from the forecast ... the use of forecast as a divisor dampens the over- forecast error … error can be more than 100%, but not accuracy. F F orecast accuracy (PA) is defined as one minus percentage error (PE), which can be expressed as PA = (1- PE) × 100% where PE = | (Actual – Forecast) | / Actual. Forecast accuracy falls between 0% (worst forecast) and 100% (perfect forecast), but PE can be greater than 100%. If PE is 100% or more, then forecast accuracy will remain at 0%. Therefore, forecast accuracy should be expressed as PA = Max {(1- PE) × 100%, 0%} Table 1 gives the percentage error and accuracy of five hypothetical SKUs (A through E). It shows that the percentage error of SKU-A is 5,400% and its accuracy is 0%. In the case of SKU-E, the percentage error is only 163% while its accuracy is still 0%. However, if the actual value is zero, then the percentage error will be infinite, but forecast accuracy will remain ...
From Issue:
Summer 2008
(Summer 2008)
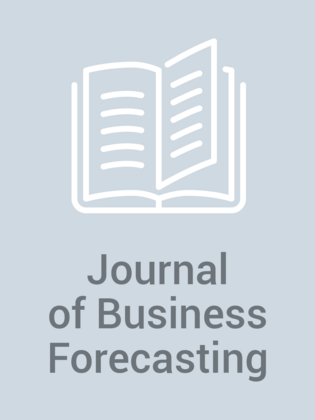