How to Manage Outliers in Demand Data Caused By Covid-19
When a black swan event occurs, our demand assumptions are suddenly thrown out the window and our historical data will no longer help generate accurate forecasts. That is challenging enough to deal with when you are in the eye of the storm, as forecasters have experienced during Covid-19. But when the dust settles, what do we do with that historical data that contains multiple outliers? In this article I reveal how to treat this data, so it doesn’t provide false demand signals for the coming periods. The methods we have at our disposal include pruning or smoothing, causal models and adjusting parameters, and manual, qualitative overrides.
From Issue:
How to Manage Outliers in Demand Data Caused By Covid-19
(Spring 2021)
IBF Journal Article by Eric Wilson, originally published in Spring 2021
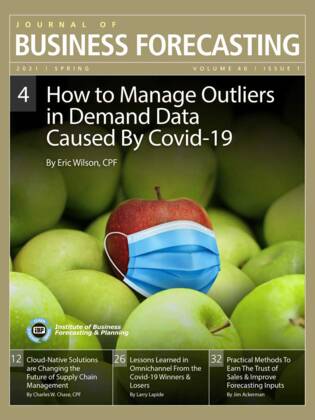
How to Manage Outliers in Demand Data Caused By Covid-19