Multi-Cluster Time Series Analysis
Most forecasting approaches are based on the idea that a forecast can be improved if the underlying factors are identified then forecasted separately. It stands to reason, then, that with conventional time series approaches that extract information from historical observations, we may gain insights by isolating and modeling elements like seasonality. In this article, I explore how transforming historical data with multi-cluster time series analysis allows us to gather additional information about seasonality. I discuss a newly introduced modelling approach that combines information from many different clusters of attributes and levels of aggregation for improved seasonal profiles, and consequently allows us to build more robust forecasts.
From Issue:
Demand Forecasting in the Cloud: Modern Computing Meets the Forecasting Discipline
(Fall 2018)
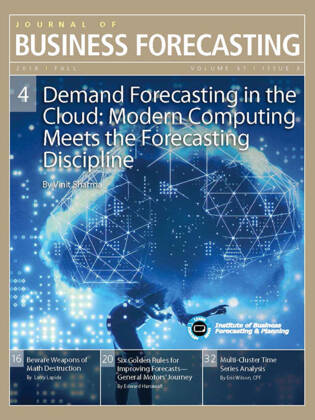