Real-Time Demand Execution Anticipating Demand at the Edge
Using real-time information as it is streaming in from connected devices on the Internet of Things (IoT), edge analytics is gaining attention as the IoT has become more widespread, streaming data from manufacturing machines, online purchases, and mobile and other remote devices. Using analytic algorithms as data are generated, at the edge of the corporate network, companies can set constraints to determine what information is worth sending to the Cloud, to a demand signal repository, or other data repositories for later use. The key benefit of edge analytics is the ability to analyze data as they are generated, which decreases latency in the decision-making process as the data are collected. Rather than designing consolidated data systems where all the data are sent back to an enterprise data warehouse (or data lake) in a raw state, where they have to be cleaned and analyzed before being of any value, why not do everything at the edge of the system, including demand forecasting, using advanced algorithms or machine learning? Are you stuck in a vicious cycle of planning demand using two-to-four-week-old data, or are you conducting real-time demand execution anticipating demand at the edge?
From Issue:
Special Issue: Future of Demand Planning & Forecasting
(Winter 2017-2018)
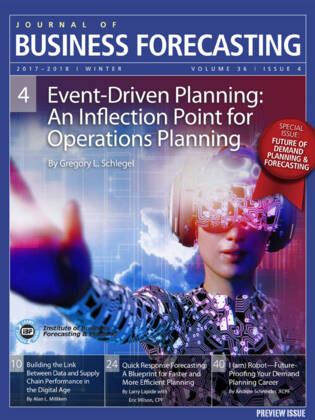